In the era of big data, emerging technologies are revolutionizing the field of data analytics, enabling organizations to harness the full potential of their data. With the evolution of data analytics and the advent of cutting-edge technologies, organizations can now extract valuable insights and make data-driven decisions like never before.
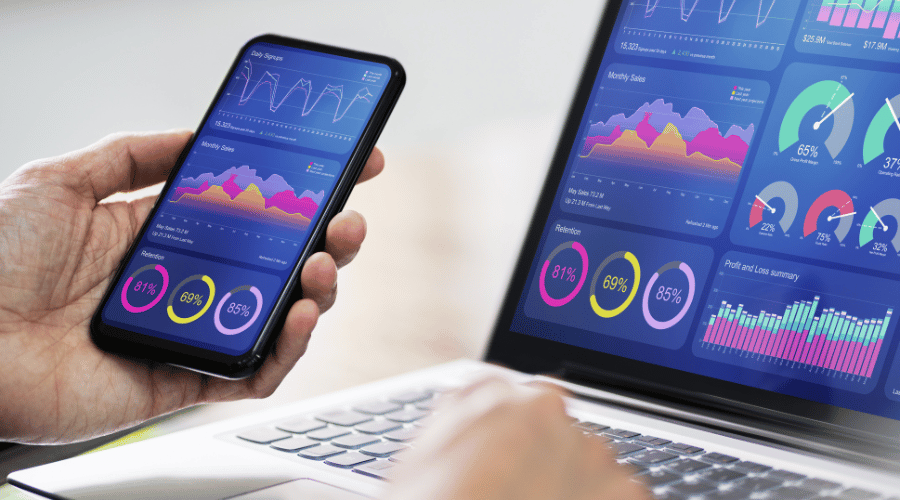
The Evolution of Data Analytics
Data analytics has come a long way, evolving from traditional approaches to leveraging cutting-edge technologies that drive insights and innovation. In the past, data analytics focused primarily on descriptive and diagnostic analytics, providing a historical view of data and identifying past patterns and trends. However, the rise of big data and the need for real-time insights have propelled the development of predictive and prescriptive analytics, which enable organizations to make accurate predictions and optimize future outcomes.
Machine Learning in Data Analytics
Machine learning, a subset of artificial intelligence, has emerged as a game-changer in data analytics, enabling organizations to uncover patterns and make accurate predictions. By using algorithms that learn from data, machine learning models can identify hidden patterns and make informed decisions without being explicitly programmed. Classification, regression, clustering, and anomaly detection are among the key applications of machine learning in data analytics. These techniques empower organizations to automate processes, detect fraudulent activities, and optimize business operations.
Deep Learning and Neural Networks
Deep learning and neural networks have revolutionized data analytics by enabling the analysis of complex and unstructured data with unprecedented accuracy. Unlike traditional machine learning algorithms, deep learning models leverage artificial neural networks with multiple layers of interconnected nodes. Convolutional neural networks (CNNs) excel in image recognition tasks, while recurrent neural networks (RNNs) are effective in processing sequential data, such as natural language processing and time series analysis. These powerful techniques have fueled advancements in image and speech recognition, language translation, recommendation systems, and more.
Natural Language Processing (NLP) and Text Analytics
Natural Language Processing (NLP) empowers data analytics by extracting valuable insights from unstructured text data, enabling organizations to leverage textual information like never before. NLP techniques enable sentiment analysis, language translation, text summarization, and chatbot development. By understanding and analyzing human language, organizations can gain deeper insights into customer feedback, social media sentiment, market trends, and more. However, challenges such as language variations, context understanding, and ethical considerations surrounding bias and privacy must be addressed for responsible and accurate NLP applications.
Edge Analytics and Real-Time Data Processing
Edge analytics and real-time data processing have become essential in the age of IoT and streaming data, enabling organizations to extract insights and make decisions in real-time. Edge analytics involves processing data closer to the source, reducing latency and bandwidth requirements. This approach is particularly valuable in scenarios where real-time decision-making is critical, such as manufacturing, healthcare, and transportation. By analyzing data at the edge, organizations can detect anomalies, optimize operations, and respond rapidly to changing conditions.
Explainable AI and Responsible Data Analytics
As data analytics becomes more complex and influential, there is a growing need for explainable AI and responsible data analytics practices. Explainable AI emphasizes the transparency and interpretability of AI models, ensuring that decisions made by algorithms can be understood and justified. This is especially crucial in sensitive domains like healthcare and finance, where decisions have significant implications. Responsible data analytics also involves mitigating bias, ensuring data privacy, and using data ethically to build trust with stakeholders and maintain societal well-being.
Unleashing the Potential: Embracing Emerging Technologies in Data Analytics
In conclusion, emerging technologies are reshaping the landscape of data analytics, providing organizations with powerful tools to unlock valuable insights and drive innovation. From machine learning and deep learning to natural language processing and edge analytics, these technologies enable organizations to make data-driven decisions, optimize processes, and gain a competitive edge. However, it is essential to embrace these technologies responsibly, addressing ethical considerations, privacy concerns, and the need for transparent and interpretable AI models. By leveraging emerging technologies in data analytics, organizations can unleash the full potential of their data and pave the way for a data-driven future.
FAQ
Q1: What is the role of machine learning in data analytics?
A1: Machine learning plays a crucial role in data analytics by enabling organizations to uncover patterns, make accurate predictions, and automate processes. It encompasses techniques such as classification, regression, clustering, and anomaly detection, empowering organizations to optimize business operations and detect fraudulent activities.
Q2: How does deep learning contribute to data analytics?
A2: Deep learning revolutionizes data analytics by utilizing artificial neural networks with multiple layers. It excels in analyzing complex and unstructured data, enabling advancements in image recognition, natural language processing, recommendation systems, and more. Deep learning enables organizations to extract valuable insights from diverse data sources.
Q3: What is the significance of natural language processing (NLP) in data analytics?
A3: Natural Language Processing (NLP) empowers data analytics by extracting valuable insights from unstructuredtext data. It enables sentiment analysis, language translation, text summarization, and chatbot development. NLP allows organizations to gain deeper insights from customer feedback, social media sentiment, and market trends, enhancing decision-making and customer engagement.
Q4: How does edge analytics facilitate real-time data processing?
A4: Edge analytics involves processing data closer to the source, reducing latency and bandwidth requirements. This approach is particularly valuable in scenarios where real-time decision-making is critical, such as manufacturing, healthcare, and transportation. By analyzing data at the edge, organizations can detect anomalies, optimize operations, and respond rapidly to changing conditions.
Q5: Why is explainable AI important in data analytics?
A5: Explainable AI emphasizes the transparency and interpretability of AI models. In sensitive domains like healthcare and finance, it is crucial to understand and justify the decisions made by algorithms. Explainable AI builds trust and enables organizations to address bias, ensure fairness, and comply with regulations.
Q6: What are the ethical considerations in data analytics?
A6: Ethical considerations in data analytics include mitigating bias, ensuring data privacy, and using data responsibly. Organizations must be mindful of potential biases in data and algorithms, protect sensitive information, and respect privacy rights. Responsible data analytics involves considering the broader societal impacts and making ethical decisions regarding data collection, usage, and sharing.
Q7: How can organizations embrace emerging technologies in data analytics responsibly?
A7: To embrace emerging technologies responsibly, organizations should prioritize transparency, interpretability, and ethical considerations. They should strive for explainable AI models, actively address biases, protect data privacy, and establish clear guidelines for data usage. Collaboration with stakeholders, adherence to regulations, and continuous monitoring and evaluation of data analytics practices are also key to responsible adoption.
Remember, as technology continues to evolve, it’s important to stay informed and adapt to emerging trends in data analytics. By understanding and harnessing the power of emerging technologies, organizations can unlock new opportunities, drive innovation, and make data-driven decisions that propel them forward in an increasingly competitive landscape.